Beyond Flat Images: Discovering Human Head Geometry with SHeaP's Self-Supervised Innovation
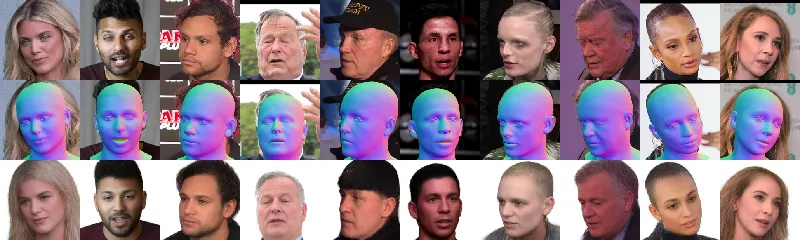
In an era where virtual reality and augmented reality are becoming increasingly prevalent, the ability to accurately reconstruct and animate 3D human heads from 2D images is revolutionizing how we interact with digital environments. Researchers have introduced an innovative technique called SHeaP (Self-supervised Head Geometry Predictor learned via 2D Gaussians), which offers a groundbreaking approach to 3D head representation by using advanced neural rendering combined with self-supervised learning.
The Challenge of 3D Reconstruction
Capturing accurate 3D models from 2D images is no simple feat. Traditional methods relied heavily on substantial 3D databases to guide the reconstruction process, which are often hard to come by and time-consuming. Moreover, previous techniques faced limitations in rendering quality and speed, primarily when capturing dynamic poses or a range of expressions. SHeaP aims to tackle these problems effectively.
A New Paradigm: Gaussian Splatting
At the heart of SHeaP is a novel approach using Gaussian Splatting. This technique represents head geometry through a combination of flexible 3D Morphable Models (3DMMs) and a series of Gaussian distributions. By integrating these two elements, SHeaP enables predictions that are not only geometrically accurate but also visually realistic. This is achieved through training on 2D videos, which enhances the model's ability to maintain fidelity in head shapes during a variety of expressions and movements.
How Does SHeaP Work?
The SHeaP methodology involves several key components. Initially, it processes a single input image to predict the 3DMM parameters—essentially the basic structure of the head—and the associated appearance Gaussians. The model reanimates a head avatar to match any target frame and adjusts based on photometric losses, improving the model's accuracy. This self-supervised strategy reduces the dependency on extensive 3D datasets and allows the model to generate cohesive 3D representations based merely on 2D data.
Achieving Unprecedented Results
In rigorous evaluations, SHeaP surpassed existing methods on benchmarks designed to test 3D geometry reconstruction accuracy, particularly excelling in the NoW benchmark for neutral faces and a newly created benchmark for diverse emotional expressions. The results highlighted not just the accuracy of head geometry but also the model's ability to capture intricate details of facial expressions, making it ideal for applications in VR, AR, and beyond.
Real-World Applications and Future Potential
The implications of SHeaP's findings extend far beyond academic interest. Accurate 3D head reconstruction is crucial for creating lifelike avatars in virtual environments, enhancing facial recognition systems, and boosting user interaction in digital media. With SHeaP's innovative architecture, the future of realistic virtual human interactions looks promising.
As researchers continue to refine this approach, we may soon see methods that can not only reconstruct heads but also incorporate full-body dynamics, emotional nuances, and environmental interactions, paving the way for richer digital experiences.