Exploring the Future of Neural Networks: The Revolutionary Miras Framework Unveiled
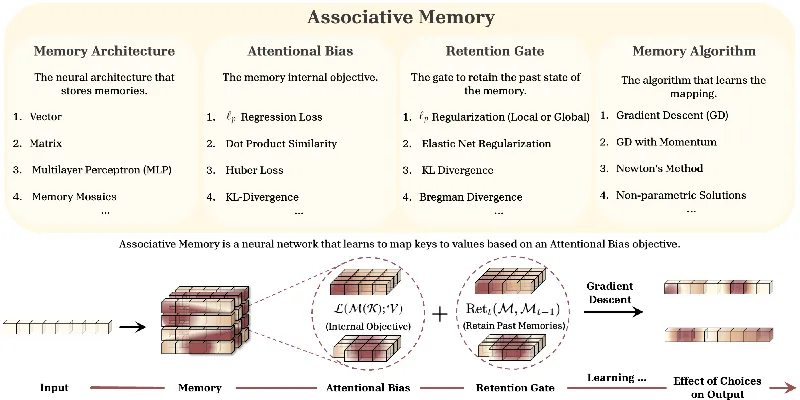
In the quest to enhance the capabilities of foundational models in artificial intelligence, researchers have introduced a groundbreaking approach in the paper titled "It’s All Connected: A Journey Through Test-Time Memorization, Attentional Bias, Retention, and Online Optimization." The authors, a team from Google Research, propose the Miras framework, which redefines how we understand neural architectures by drawing inspiration from human cognitive phenomena, particularly attentional bias.
The Core Concept: Associative Memory
At the heart of this research is the concept of associative memory. The authors argue that modern neural networks—including popular models like Transformers—can be viewed as sophisticated associative memory modules. This perspective not only highlights the connection between input and output through keys and values but also introduces a novel internal objective known as attentional bias.
Attentional bias allows these networks to prioritize certain inputs over others, resembling how humans instinctively focus on significant stimuli in overwhelming environments. This framing sets the stage for understanding how machines can learn and "remember" more effectively.
Unpacking Miras: Configurations and Innovations
The Miras framework simplifies the design of neural architectures by presenting four core choices: memory architecture, attentional bias objective, retention gate, and memory learning algorithm. Each of these elements plays a crucial role in determining how effectively a model can manage its understanding of context and information over time.
The authors introduce three innovative sequence models—Moneta, Yaad, and Memora—tailored to leverage the strengths of Miras. These models demonstrate a significant performance edge in various tasks, including language modeling and commonsense reasoning, outperforming established architectures like Transformers.
Technical Advances Simplified
While the research includes complex mathematical formulations and technical jargon, the essence lies in its practicality. Essentially, Miras allows for a more efficient training process by effectively managing memory and adaptation during task execution. The choice of attentional bias, whether it’s a simple dot-product similarity or a more complex ℓ_p norm, can drastically influence how well a model learns from and retains information.
Moreover, the introduction of retention gates—a method to balance the learning of new information with the retention of previously acquired knowledge—presents a more humane approach to AI memory systems. This mechanism parallels human memory processes, making AI models not just faster but also smarter in their learning capabilities.
Implications for the Future
The implications of Miras are profound. As artificial intelligence continues to integrate into our daily lives, the need for models that mimic human cognition becomes increasingly important. This framework doesn't just enhance performance; it sets a precedent for how future models can be designed to think, learn, and adapt more naturally.
With promising results reported in varied tasks, Miras might just lead the next wave of breakthroughs in AI research, ensuring that as models evolve, they do so with memory mechanisms that reflect our own cognitive processes.