Mapping the Future: How LatentAct Transforms 3D Hand Interactions from Simple Images
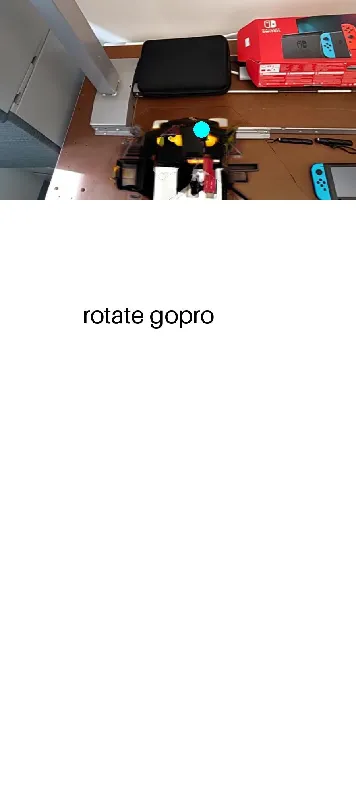
In an era where artificial intelligence plays an ever-growing role in our daily lives, a recent research paper titled “How Do I Do That? Synthesizing 3D Hand Motion and Contacts for Everyday Interactions” unveils a groundbreaking approach to predicting hand movements and interactions with everyday objects. This innovative method, developed by a team of researchers from the University of Illinois Urbana-Champaign and Microsoft, could significantly enhance various applications from robotics to virtual reality.
The Challenge of Predicting Hand Interactions
Hand movements are complex and can vary greatly depending on the task at hand. For example, the technique used to screw in a bolt is distinct from that used to pour coffee. The researchers identified a challenge: how to accurately predict the trajectories of hand movements and the points of contact with objects using very minimal initial information such as a single image and an action description.
The solution they present, dubbed LatentAct, involves a two-pronged strategy. First, they created an “Interaction Codebook” that allows the system to learn and tokenize various hand poses and the associated contact points through an advanced model. Then, an “Interaction Predictor” utilizes this codebook to forecast the hand's movements based on given inputs.
Unpacking the Methodology
At the heart of LatentAct is a sophisticated model architecture that leverages a Visual-Quantized Variational Autoencoder (VQVAE) coupled with a transformer-decoder mechanism. This enables the system to distill hand motion into a library of unique interaction trajectories, from which the model can draw when predicting future movements.
Data plays a crucial role in this model's effectiveness. The researchers built a comprehensive dataset by analyzing over 2,000 egocentric videos demonstrating a variety of normal human-object interactions. This dataset is 2.5 to 10 times more diverse than existing resources, incorporating numerous tasks and object categories.
Impressive Performance and Generalization
The researchers conducted extensive experiments to validate LatentAct's efficacy, measuring its performance across several factors such as novel objects, actions, and scenes. The results reflected a significant improvement in accuracy over traditional methods, demonstrating LatentAct's ability to generalize well to various scenarios, including some where initial hand information could not be seen in the input image.
By producing more accurate predictions of 3D hand positions and contact maps, LatentAct is set to revolutionize fields requiring precise hand-object interactions, making it a noteworthy advance in computer vision and robotics.
The Future of Human-Object Interaction
As we look ahead, the potential applications for LatentAct are vast. From enhancing user interfaces in virtual reality to enabling more adept robots capable of manipulating objects in our everyday environment, the implications of this research are profound. By bridging the gap between image data and hand interaction synthesis, LatentAct paves the way for new innovations that could redefine our engagement with technology.
The endeavor represents an exciting step towards more intuitive and responsive AI systems, reinforcing the importance of interdisciplinary collaboration in tackling complex challenges presented by human-like interactions in machines.