Revolutionizing Cross-Modal Retrieval: How SemCORE is Redefining Semantic Understanding
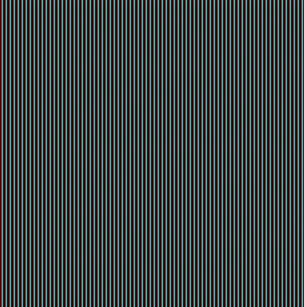
In today's digital age, cross-modal retrieval (CMR)—the process of finding relevant information across different data types like text and images—is crucial for effective multimedia research. A recent study led by a team from various universities introduced a groundbreaking framework called SemCORE, which enhances this retrieval process through advanced semantic understanding. Unlike traditional methods that rely heavily on static indexing, SemCORE leverages the potency of generative models to greatly improve the accuracy and efficiency of cross-modal retrieval.
Understanding the Core Challenges in Cross-Modal Retrieval
Conventional CMR methods often involve significant computational overhead as they compare texts and images based solely on numeric identifiers. This technique not only raises latency issues when datasets grow but also frequently suffers from semantic misinterpretation, where the tasks of identifying and retrieving relevant content lag behind current technological capabilities. The study identifies two major limitations: the insufficient semantic richness of identifiers and a lack of fine-grained semantic differentiation in retrieval outcomes.
Introducing SemCORE: A Semantic Enhancement Strategy
SemCORE addresses these challenges head-on by creating a unified framework that combines Structured natural language Identifiers (SID) and a Generative Semantic Verification (GSV) strategy. The SID is ingeniously composed of two components: a Global ID for overarching semantic categories and a Lexical ID focusing on detail-rich, contextually relevant keywords. This approach not only enriches the semantic texture of the identifiers but also empowers generative models to perform more accurately in matching queries to relevant visual or textual data.
Key Innovations in SemCORE
One of the standout features of SemCORE is its dual focus on both text-to-image and image-to-text retrieval tasks, a departure from previous models that typically focused on one direction. The GSV further refines this functionality by employing fine-grained analyses to distinguish between similar targets, making retrieval both precise and efficient.
Experimental results have shown that SemCORE significantly outperforms existing generative techniques, achieving an impressive average of 8.65 points improvement in Recall@1 for text-to-image searches. This metric is particularly vital in assessing how often the first retrieved item matches the desired result, highlighting SemCORE's effectiveness at providing relevant and timely results.
The Path Forward for Generative Retrieval
By harnessing the power of multi-modal large language models (MLLMs), SemCORE not only enhances retrieval performance but also sets a roadmap for future developments in generative retrieval. The authors of the study express optimism in further exploring dynamic retrieval as real-world datasets evolve, indicating that improving the generalization capabilities of generative models will be a key focus area.
In conclusion, the introduction of SemCORE represents a significant step toward revolutionizing cross-modal retrieval, merging semantic understanding with generative capabilities to create an efficient and effective multimedia research tool. As the field continues to evolve, solutions like SemCORE may pave the way for even more intelligent systems capable of interpreting and retrieving information across various data types seamlessly.