Revolutionizing Personalized Image Creation: Auto-Regressive Models Take Center Stage
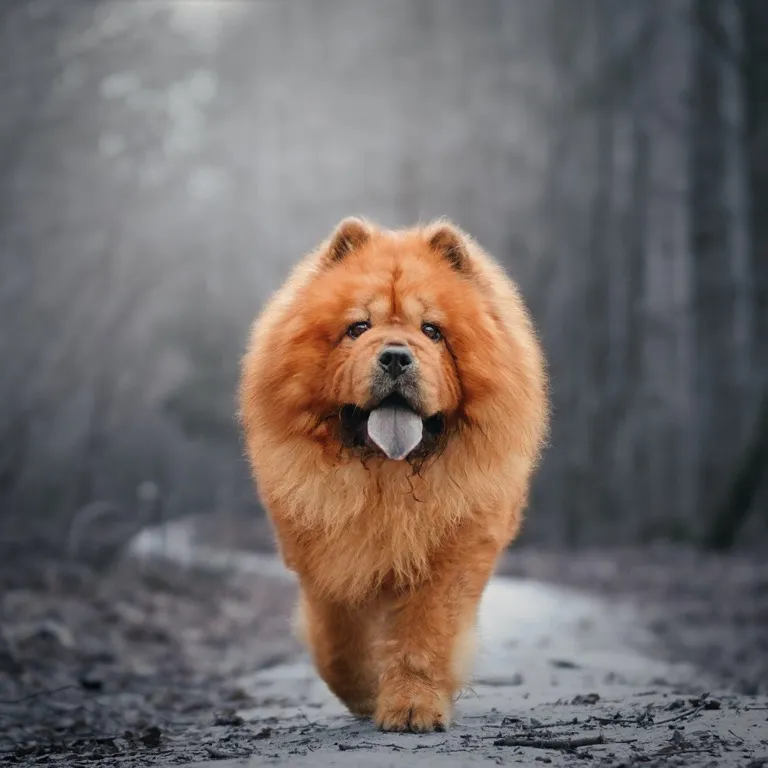
The landscape of personalized image synthesis is rapidly evolving, and a recent study sheds light on an innovative two-stage training technique for improving auto-regressive models in text-to-image generation. Conducted by researchers Kaiyue Sun, Xian Liu, Yao Teng, and Xihui Liu, this groundbreaking research highlights the ability of auto-regressive models to generate highly customized images using a limited amount of reference images.
Understanding the Shift from Diffusion Models
For quite some time, diffusion models have dominated the field of personalized image generation. These models excel at producing realistic images by iteratively refining hints from the data. However, the researchers note that auto-regressive models, which generate images by predicting a sequence of tokens, have remained an underexplored territory for personalizing image creation. The study emphasizes that these models can leverage multimodal capabilities, offering a fresh perspective on synthetic image generation.
A New Approach: Two-Stage Training Strategy
The authors propose a novel two-stage training strategy aimed at enhancing the efficiency and fidelity of personalized image synthesis. The first stage focuses on optimizing text embeddings, which are mathematical representations that correspond to specific subjects. Once this is accomplished, the second stage fine-tunes the transformer layers of the auto-regressive model to refine the generated outputs. This method not only stabilizes training but significantly boosts the model’s ability to generate images that accurately reflect the intended subject, a feat that the researchers demonstrated through rigorous experimentation on the Lumina-mGPT 7B model.
Impressive Results and Comparisons
In tests comparing their approach to existing methods like Textual Inversion and DreamBooth, the researchers found that their auto-regressive model achieved comparable fidelity and prompt-following accuracy while excelling in prompt alignment. Generated images displayed a robust understanding of various subjects, affirming the model's capacity for capturing and reproducing unique characteristics. The research results indicate that utilizing just 3-5 images for training can produce remarkably personalized visuals, pointing toward a promising future for customizable image generation technologies.
Potential Applications and Ethical Considerations
This advancement in personalized image creation has significant implications for various domains, including digital art, advertising, and virtual reality. However, the study also acknowledges potential challenges associated with such powerful capabilities, particularly regarding the misuse of generated images to create misleading content. The authors stress the need for ethical guidelines and responsible advancements in generative technologies, a concern that is becoming increasingly relevant as these models proliferate.
In conclusion, the study highlights a pivotal moment in the evolution of personalized image synthesis, suggesting that auto-regressive models may offer a viable and highly effective alternative to diffusion-based approaches. As researchers continue to refine this technology, the possibilities for creative applications are vast—and the implications warrant thoughtful consideration.