Revolutionizing Video Game AI: The Quest for Perfect Trajectory Following in 3D Worlds
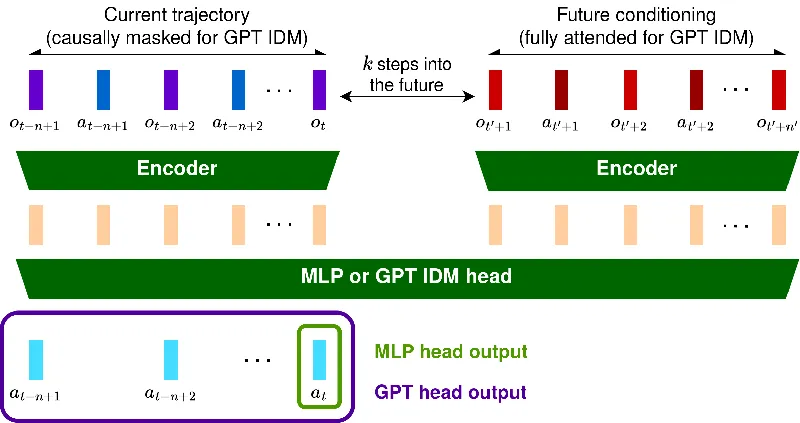
Researchers have taken a significant step in the field of artificial intelligence and video gaming with their recent study on trajectory following in 3D environments. Published as a workshop paper at ICLR 2025, the work investigates how agents can imitate expert players in complex video games, specifically focusing on a modern 3D game titled Bleeding Edge.
Understanding the Challenge
Imitation learning has proven to be a powerful tool for training agents using expert knowledge. However, in dynamic environments like video games, challenges such as distribution shifts and stochasticity complicate the process. In simple terms, these complexities mean that a game AI can't simply replay past actions—things can change dramatically with each play-through. The researchers in this study sought to adapt Inverse Dynamics Models (IDM) to enhance trajectory replication, which is crucial to imitating human-like player behavior.
Innovative Techniques and Approaches
The study emphasizes the use of various encoders and policy heads to create models capable of accurately following trajectories. The authors introduced three types of encoders: a game-specific one suited for Bleeding Edge, a general-purpose encoder, and a model trained from scratch. By exploring different configurations, the team aimed to identify the most effective combination for both low and high data settings.
One of the critical findings is that using a GPT-style policy head combined with a newly trained encoder yields promising results in diverse data settings. Conversely, when data is limited, pairs combining a DINOv2 encoder and a GPT-style head showed optimal performance. In short, the study highlights that the right model configuration can significantly reduce trajectory deviations in the game.
The Future of Imitation Learning
The implications of this research extend beyond gaming. The findings suggest that reinforcement learning techniques, refined through innovative models like IDM-K, can be widely applicable in fields ranging from robotics to autonomous vehicles. With AI's growing capabilities to learn from human-like behavior, the potential for advanced applications is immense.
The researchers emphasized that while advancements have been made, much work remains before trajectory-following can be perfected in various gameplay contexts. As models continue to improve in robustness against unexpected changes and allow for nuanced human-like interactions, the gaming experience—and potentially many other domains—could reach new heights of realism and adaptability.