Transforming Robot Learning: How RoboSplat is Redefining Training with 3D Gaussian Splatting
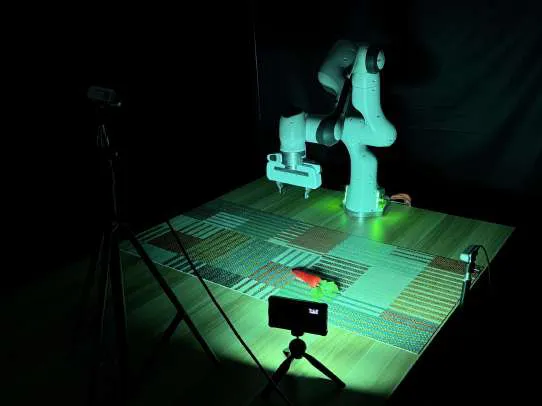
In the rapidly evolving field of artificial intelligence, robotic manipulation stands out as a challenging frontier. A recent research paper introduces a groundbreaking approach called RoboSplat, which enhances robot training efficiency by employing 3D Gaussian Splatting to generate diverse and realistic demonstrations from a single expert maneuver. This innovation significantly improves the performance of robots in real-world tasks, allowing them to adapt to various environments with minimal data input.
The Challenge of Traditional Training Methods
Traditional methods for training robotic manipulation systems rely heavily on the collection of extensive datasets through human demonstrations. This process is not only labor-intensive but also time-consuming, often requiring hundreds of hours of footage to achieve robust results. Moreover, the existing augmentation techniques mainly focus on 2D data, which can lead to spatial inaccuracies and hinder a robot's ability to generalize across different tasks and settings.
Unveiling RoboSplat: A Game-Changer in Robotic Training
RoboSplat leverages the power of 3D Gaussian Splatting (3DGS) to reconstruct scenes in high fidelity, allowing robots to learn more effectively from minimal input. The essence of this technique resides in its ability to represent complex scenes with a collection of 3D Gaussian clouds, enabling precise manipulation and data augmentation. By generating new demonstrations based on a single expert trajectory, RoboSplat can enrich the training data across multiple aspects such as object types, poses, and environmental conditions.
Key Innovations and Outcomes
The research introduces several innovative strategies that enhance how robots learn through augmentation:
- 3D Gaussian Replacement: This technique allows for the substitution of objects within the training scene, providing the robot with varied experiences that mirror real-world conditions.
- View Synthesis: By enabling novel camera perspectives, this method allows robots to learn from diverse observational angles, crucial for tasks where the view might differ from the initial training setup.
- Scene Appearance Adjustment: By altering lighting and background elements, the robot learns to adapt to different environments, improving its robustness in handling real-world scenarios.
Experiments conducted as part of the study demonstrate that robots trained with RoboSplat not only surpassed their traditionally trained counterparts but did so with an impressive success rate of 87.8% in one-shot settings against the standard 57.2%. This remarkable increase underscores the effectiveness of the approach, ensuring that even minimal training data can yield high-performance outcomes.
Real-World Implications and Future Directions
The implications of RoboSplat are vast, potentially revolutionizing how robotic systems are trained for complex tasks such as pick-and-place operations, tool handling, and long-horizon strategies. Furthermore, the flexibility of the technique opens up avenues for future research to incorporate more dynamic and complex environments, including interactions with deformable objects and real-time adaptability.
As the field of robotics grows ever more intertwined with artificial intelligence, innovations like RoboSplat represent significant strides not just in the technology’s capabilities but in its accessibility and applicability in real-world situations. With continued advancements, the dream of fully adaptable, independent robotic systems is moving closer to reality.