Transforming Vision-Language Models: How REVERSE Reduces Hallucination Risks by Self-Correcting Errors
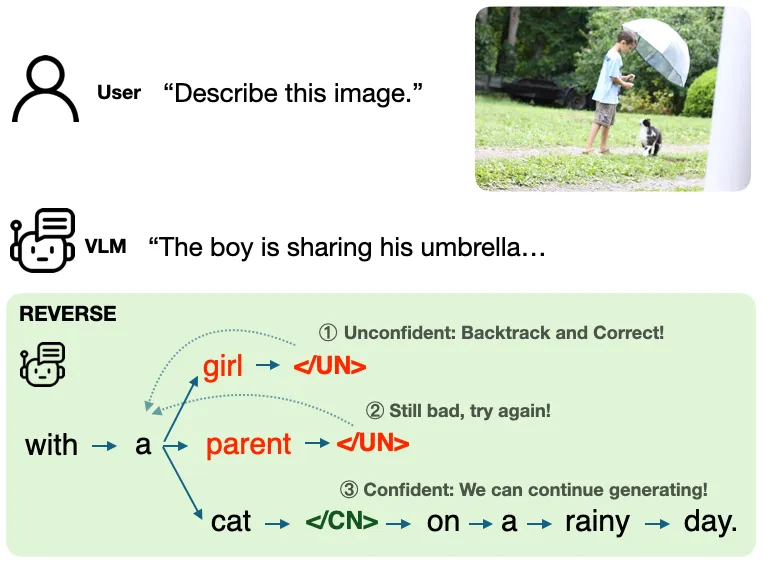
In the ever-evolving field of artificial intelligence, Vision-Language Models (VLMs) have emerged as powerful tools for tasks like visual question answering and image captioning. However, they often generate misleading outputs known as visual hallucinations—descriptions of objects or actions that don’t exist. A recent study from researchers at UC Berkeley introduces a groundbreaking method called REVERSE that not only detects these hallucinations but also enables the models to self-correct in real-time during the generation process.
The Problem of Visual Hallucination
Visual hallucinations in VLMs can have critical implications, particularly in safety-sensitive applications such as autonomous driving and assistive technologies for the visually impaired. The existing strategies to combat this issue typically fall into two categories: generation adjustment, which modifies the output based on visual data, and post-hoc verification, which uses separate models to correct outputs after they are generated. While both methods show promise, they have significant drawbacks, such as relying on heuristics or suffering from error propagation.
Introducing REVERSE: A New Framework
The researchers propose REVERSE (Retrospective Verification and Self-Correction)—a novel framework that combines these two methods into a single architecture. By leveraging a specially constructed dataset of over 1.3 million semi-synthetic samples, REVERSE trains VLMs to tag potential hallucinations during the generation phase. This allows the model to dynamically evaluate and refine its output as it generates text.
Key Innovations in the REVERSE Framework
One of the central mechanisms of REVERSE is a process called retrospective resampling. During output generation, the model can flag a phrase as potentially hallucinated—indicated by a special token. If the probability of this token exceeds a predefined threshold, the model automatically backtracks to a previous point in the output and attempts to regenerate a more accurate phrase, effectively correcting its own mistakes without needing external verification.
The framework's architecture includes three special tokens to help classify generated phrases: one denotes the start of a key phrase, another signals a confident or grounded phrase, and the last indicates a hallucinated phrase. This tokenization empowers the model to critically assess its own outputs continuously.
Results and Evaluations
In extensive evaluations across various benchmarks, REVERSE has demonstrated substantial improvements. The findings show up to a 12% reduction in hallucinations on the CHAIR-MSCOCO benchmark and a remarkable 28% reduction on the HaloQuest benchmark compared to the best existing methods. These results position REVERSE as a formidable advancement in addressing the hallucination limitations of VLMs.
Conclusion and Future Directions
By integrating self-verification capabilities directly into VLMs, REVERSE not only enhances model accuracy but also establishes new standards for how AI systems can manage and mitigate hallucinations during output generation. Future research may focus on further refining these self-correcting mechanisms and applying similar frameworks to other domains within multimodal AI, thus paving the way for more reliable and trustworthy AI systems.