Unlocking the Hidden Power of Perception Encoder: Why Intermediate Layers Might Be Your Best Bet
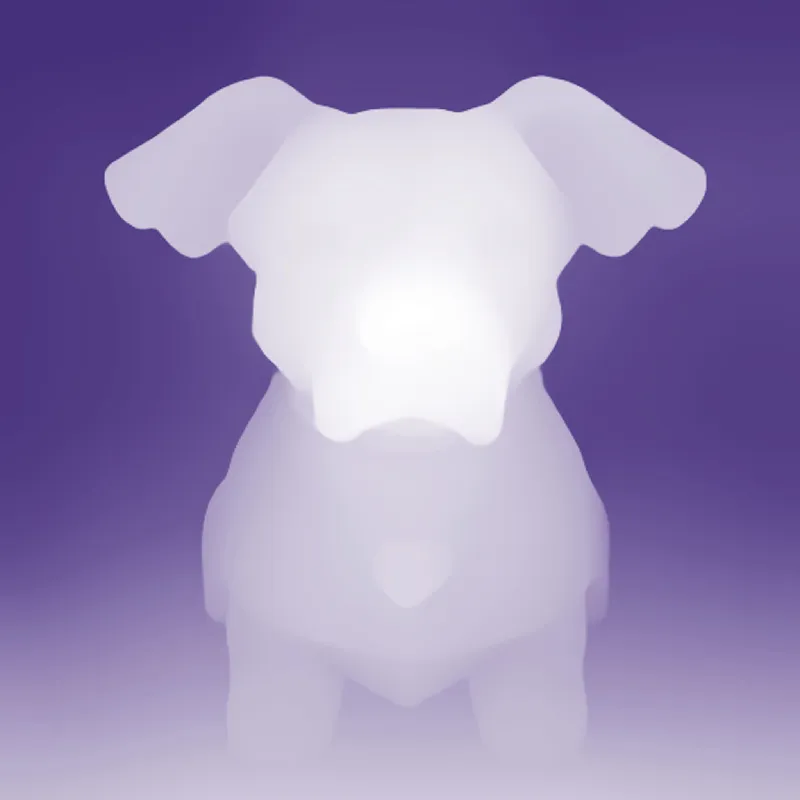
Recent research from a team at Meta FAIR has introduced an innovative model known as the Perception Encoder (PE), which challenges conventional wisdom in image and video understanding. By harnessing the power of contrastive vision-language training, PE reveals that some of the most valuable visual embeddings lie not at the network's output, but within its intermediate layers. This finding is set to revolutionize how we approach tasks like classification and retrieval across a variety of applications.
What is the Perception Encoder (PE)?
The Perception Encoder (PE) is a state-of-the-art encoder designed specifically for image and video understanding. Unlike traditional encoders, which utilize various pretraining objectives for specific tasks—such as classification or localization—PE employs a simplified approach through robust contrastive vision-language training. This methodology allows the model to generate powerful global embeddings suitable for numerous downstream tasks.
Surprising Findings: The Strength of Intermediate Layers
One of the standout revelations from the research is that while many might expect the most effective features from a model to reside in its final layers, PE demonstrates otherwise. It turns out that the hidden layers of the network contain specific features that align closely with a range of tasks like object detection, visual question answering, and even tracking. This means that the ideal embeddings for various applications are often embedded deep within the model, waiting to be extracted and utilized effectively.
Harnessing the Power with Alignment Techniques
To access the latent features housed in these intermediate layers, the researchers introduced two alignment methods: language alignment and spatial alignment. The language alignment approach effectively bridges these features to a language model, thereby enhancing multimodal language modeling tasks. Conversely, spatial alignment focuses on adapting the visual characteristics of these intermediate features, ensuring they lend themselves to high-performance object detection and other spatial tasks.
State-of-the-Art Performance on Multiple Tasks
PE not only excels in identifying and retrieving images and videos through zero-shot classification, but also outperforms previous models trained on larger datasets. Documentation from the research indicates that PE achieves a remarkable level of robustness across various benchmarks, solidifying its position as a comprehensive solution for visual task performance.
Why This Matters
The implications of such a model are profound. PE opens new avenues for improving technology in areas such as autonomous driving, content moderation, and even interactive AI applications by providing more accurate and adaptable visual processing capabilities. The insights gathered from this research are expected to drive future developments in multimodal models and applications that require sophisticated visual understanding.
As the field of computer vision continues to evolve, the Perception Encoder stands as a pivotal advancement, illustrating how novel training techniques and deeper insights into model architecture can lead to groundbreaking results in understanding visual data.