Unveiling the Future of Visual Understanding with fPerceptionLM: A Game-Changer in Open-Access AI
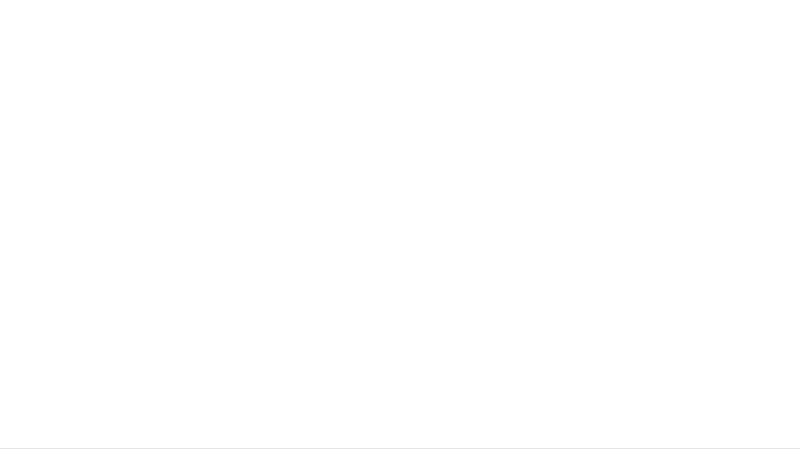
In the ever-evolving world of artificial intelligence, the recent research paper titled "fPerceptionLM: Open-Access Data and Models for Detailed Visual Understanding" presents an electrifying breakthrough that holds the potential to transform how we approach computer vision tasks. Authored by a collaborative team from Meta FAIR and UT Austin, this research offers a fully open and reproducible framework aimed at enhancing our understanding of images and videos.
The Challenge of Closed-Source Models
Vision-Language Models (VLMs) have become essential tools for researchers and developers, but many top-performing models remain cloaked in mystery due to their closed-source nature. This secrecy hinders reproducibility and leaves researchers struggling to measure genuine scientific progress. Often, advancements in benchmark results stem from merely distilling information from these black-box models, without fostering innovation in model design or training methods.
Introducing the Perception Language Model (PLM)
The authors introduced the Perception Language Model (PLM), a pioneering initiative designed to address the gaps brought about by proprietary systems. By focusing on a fully transparent approach, the research team has released a staggering 2.8 million human-labeled instances of fine-grained video question-answer pairs and detailed video captions, filling critical data gaps in the AI visual understanding space. This release not only enhances the model's capabilities but also sets a new benchmark for open-access datasets.
Why Human-Annotated Data Matters
One of the standout features of the PLM is its robust dataset, comprising both synthetic and human-annotated data. While synthetic data allows for scaling up training quickly, it often lacks the nuanced understanding needed for real-world applications. The introduction of human-annotated data addresses this shortfall, enabling the development of models capable of performing detailed reasoning about visual content and complex activities portrayed in videos.
PLM–VideoBench: A Step Forward for Benchmarking
Moreover, the research introduces PLM–VideoBench, a specialized benchmarking suite designed to evaluate the performance of VLMs on challenging video understanding tasks. This benchmark emphasizes the ability of AI to reason across various dimensions of visual media, including "what," "where," "when," and "how" events are represented.
Setting New Standards in Visual Understanding
Through rigorous evaluation across 40 image and video benchmarks, PLM demonstrates performance that rivals existing state-of-the-art models, even outperforming certain proprietary systems on key tasks. The implications of this research are monumental, as it paves the way for greater collaboration within the AI research community and promises a brighter future for reproducible research.
In conclusion, the fPerceptionLM paper is a call to action for researchers to embrace open-access methodologies, offering a glimpse into a future where AI can understand complex visual environments with unprecedented detail. As the boundaries of technology are pushed forward, tools like PLM stand to redefine our relationship with visual content and enhance our capabilities in AI-driven analysis.