Unveiling the Underlap Coefficient: A Breakthrough in Diagnosing Alzheimer's Disease Biomarkers
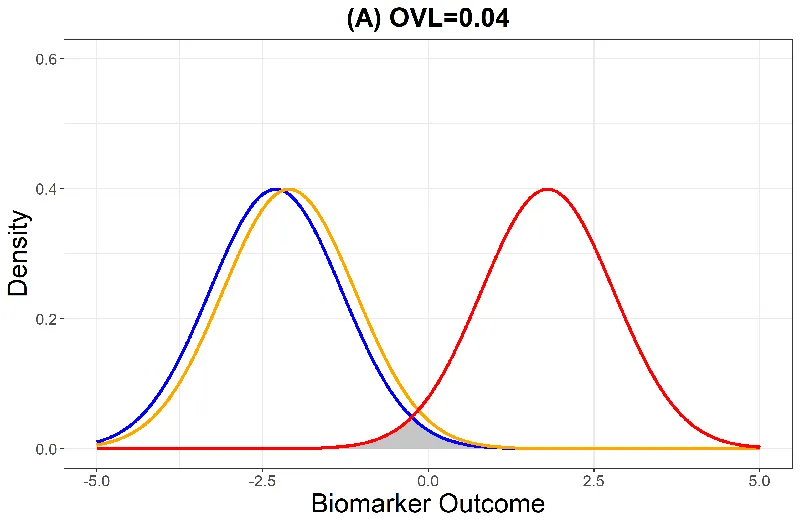
In the realm of biomedical research, accurately distinguishing between various disease stages is pivotal, particularly in conditions like Alzheimer's disease (AD). A recent scholarly article introduces a groundbreaking statistical approach known as the underlap coefficient, which promises to enhance the discriminatory power of biomarkers used in diagnosing AD. This innovative metric challenges traditional assumptions and offers a more versatile tool in understanding how biomarkers function across different disease states.
The Need for Better Biomarker Evaluation
Evaluating the efficacy of biomarkers – measurable indicators of the severity or presence of a disease – is crucial for early diagnosis and treatment. Up until now, measures such as the area under the receiver operating characteristic surface (AUC) were prominent. However, these conventional methods rely on stochastic ordering assumptions, which can pose limitations, especially when assessing multiple disease classes. When these assumptions are violated, they lead to potentially misleading conclusions about a biomarker's effectiveness.
Introducing the Underlap Coefficient
The authors propose the underlap coefficient (UNL) as a more adaptable and intuitive measure. Unlike previous models, the UNL does not depend on any specific distribution order and can evaluate biomarkers' performance across three or more conditions without assuming that greater values reflect more severe disease stages. This flexibility allows for a broader application across diverse biomarker scenarios, including instances where lower values might indicate serious conditions.
How the Underlap Coefficient Works
The UNL quantifies the degree of separation between different biomarker distributions. It ranges from one to three—where one signifies complete overlap of biomarker outcome distributions and three indicates perfect separation. This mathematical construct aids in effectively summarizing the distinctions among varying disease states, thus serving as a valuable diagnostic tool.
A Practical Application: Alzheimer's Disease
The research paper illustrates the UNL's application using a dataset from the Alzheimer’s Disease Neuroimaging Initiative (ADNI), focusing on biomarkers such as cerebrospinal fluid (CSF) Abeta, Tau, and phosphorylated tau (pTau). By examining how these biomarkers differentiate between cognitively normal individuals, those with mild cognitive impairment, and patients with full-blown Alzheimer’s, the study uncovers the nuanced performance characteristics of these indicators in varied demographic groups.
Implications for Future Research and Practice
The promising results indicate that the UNL could significantly streamline the evaluation process of potential biomarkers in clinical settings, particularly in the context of complex diseases like Alzheimer's. By allowing researchers to account for variations in patient characteristics, such as age and gender, the underlap coefficient enhances the precision in assessing how well these biomarkers can ultimately differentiate the stages of Alzheimer’s, paving the way for improved diagnostic protocols.
In conclusion, the introduction of the underlap coefficient marks a significant advancement in the statistical methodologies used within the medical research community. As scientists and clinicians seek to enhance the tools available for diagnosing Alzheimer's and other multi-stage diseases, the UNL stands out as a promising framework that might just redefine how pathology is understood and treated.